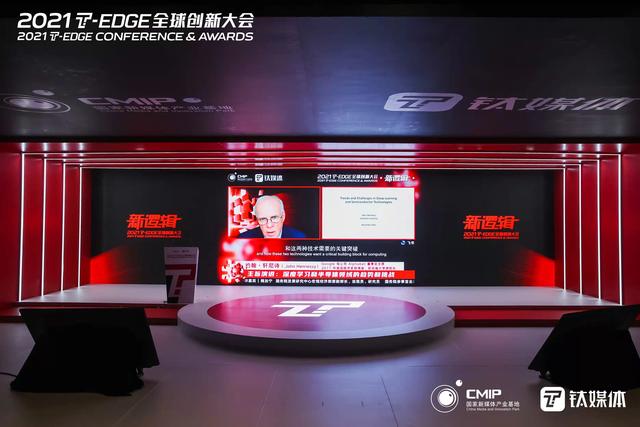
50年前,英特尔创始人之一戈登·摩尔提出了摩尔定律:集成电路上可容纳的晶体管数目,约每隔24个月便会增加一倍。但近两年,关于摩尔定律是否失效的争论不断。
与摩尔定律(Moore’s law)相伴而生的是登纳德缩放定律(Dennard scaling),即随着晶体管密度的增加,每个晶体管的功耗会下降,因此每平方毫米硅的功耗几乎是恒定的。但登纳德缩放定律在2007年开始显著放缓,到2012年几乎失效。
也就是说,半导体技术的更新迭代显然已经无法带来飞跃的性能增长,即使多核设计也没有显著改善能效方面的问题。这种情况下,能否找到更加高效的利用方法?未来半导体行业又会出现哪些变化趋势?
围绕这一问题,在钛媒体和国家新媒体产业基地联合主办的2021 T-EDGE全球创新大会上,Google母公司Alphabet董事会主席、2017年美国图灵奖获得者、斯坦福大学原校长John Hennessy发表了题为《深度学习和半导体技术领域的趋势和挑战》演讲。
在他看来,实现更高的性能改进需要新的架构方法,从而更有效地使用集成电路功能。具体的解决方案有三个可能的方向:
1、以软件为中心的机制。即着眼于提高软件的效率,以便更有效地利用硬件;
2、以硬件为中心的方法。也称为特定领域架构或特定领域加速器;
3、以上两类的部分结合。开发出与这些特定架构相匹配的语言,让人们更有效地开发应用程序。
在这样的变化之下,John Hennessy认为,“未来通用处理器将不是驱动行业发展的主力,能够与软件快速联动的特定领域处理器将会逐渐发挥重大作用。因此,接下来或许会看到一个更垂直的行业,会看到拥有深度学习和机器学习模型的开发者与操作系统和编译器的开发者之间更垂直的整合,使他们的程序能够有效运行、有效地训练以及进入实际使用。”
以下为John Hennessy演讲实录,经钛媒体编辑整理:
Hello I’m John Hennessy, professor of computer science and electrical engineering at Stanford University, and co-winner of the Turing Award in 2017.
大家好,我是约翰·轩尼诗,斯坦福大学计算机科学与电气工程教授,也是2017 年图灵奖共同获得者。
It’s my pleasure to participate in the 2021 T-EDGE conference.
很高兴能参加 2021年的 T-EDGE 大会。
Today I’m going to talk to you about the trends and challenges in deep learning and semiconductor technologies, and how these two technologies want a critical building block for computing and the other incredible new breakthroughs in how we use computers are interacting, conflicting and how they might go forward.
今天我想谈谈深度学习和半导体技术领域的趋势和挑战、这两种技术需要的关键突破、以及计算机领域的其他重大突破和发展方向。
AI has been around for roughly 60 years and for many years it continues to make progress but at a slow rate, much lower than many of the early prophets of AI had predicted.
人工智能技术已经存在大约 60 年,多年来持续发展。但是人工智能技术的发展开始放缓,发展速度已远低许多早期的预测。
And then there was a DRAMatic breakthrough around deep learning for several small examples but certainly AlphaGo defeating the world’s go champion at least ten years before it was expected was a dramatic breakthrough. It relied on deep learning technologies, and it exhibited what even professional go players would say was creative play.
在深度学习上我们实现了重大突破。最出名的例子应该就是 AlphaGo 打败了围棋世界冠军,这个成果要比预期早了至少十年。Alpha Go使用的就是深度学习技术,甚至连专业人类棋手也夸赞Alpha Go的棋艺颇具创意。
That was the beginning of a world change.
这是巨变的开端。
Today we’ve seen many other deep learning breakthroughs where deep learning is being used for complex problems, obviously crucial for image recognition which enables self-driving cars, becoming more and more useful in medical diagnosis, for example, looking at images of skin to tell whether or not a lesion is cancerous or not, and applications in natural language particularly around machine translation.
今天,深度学习也在其他领域取得重大突破,被应用于解决复杂的问题。其中最明显的自然是图像识别技术,它让自动驾驶技术成为可能。图像识别技术在医学诊断中也变得越来越有用,可通过查看皮肤图像判断是否存在癌变。除此之外,还有在自然语言处理中的应用,尤其是在机器翻译方面颇具成果。
Now for Latin-based language basically being as good as professional translators and improving constantly for Chinese to English, a much more challenging translation problem but we are seeing even a significant progress.
目前,拉丁语系的机器翻译基本上能做到和专业翻译人员相似的质量。在更具挑战的汉英翻译方面上,机器翻译也有不断改进,我们已经能看到显着的进步。
Most recently we’ve seen AlphaFold 2, a deep minds approach to using deep learning for protein folding, which advanced the field by at least a decade in terms of what is doable in terms of applying this technology to biology and going to dramatically change the way we make new drug discovery in the future.
近期我们也有 AlphaFold 2,一种使用深度学习进行蛋白质结构预测的应用,它将深度学习与生物学进行结合,让该类型的应用进步了至少十年,将极大程度地改变药物研发的方式。
What drove this incredible breakthrough in deep learning? Clearly the technology concepts have been around for a while and in fact many cases have been discarded earlier.
是什么让深度学习取得了以上突破?显然,这些技术概念已经存在一段时间了,在某种程度上也曾被抛弃过。
So why was it able to make this breakthrough now?
那么为什么现在我们能够取得突破呢?
First of all, we had massive amounts of data for training. The Internet is a treasure trove of data that can be used for training. ImageNet was a critical tool for training image recognition. Today, close to 100,000 objects are on ImageNet and more than 1000 images per object, enough to train image recognition systems really well. So that was the key.
首先是我们有了大量的数据用于训练AI。互联网是数据的宝库。例如 ImageNet ,就是训练图像识别的重要工具。现在ImageNet 上有近 100,000 种物体的图像,每种物体有超过 1000 张图像,这足以让我们很好地训练图像识别系统。这是重要变化之一。
Obviously we have lots of other data were using here for whether it’s protein folding or medical diagnosis or natural language we’re relying on the data that’s available on the Internet that’s been accurately labeled to be used for training.
我们当然也使用了其他大量的数据,无论是蛋白质结构、医学诊断还是自然语言处理方面,我们都依赖互联网上的数据。当然,这些数据需要被准确标记才能用于训练。
Second, we were able to marshal mass of computational resources primarily through large data centers and cloud-based computing. Training takes hours and hours using thousands of specialized processors. We simply didn’t have this capability earlier. So that was crucial to solving the training problem.
第二,大型数据中心和云计算给我们带来了大量的运算资源。使用数千个专用处理器进行人工智能训练只需要数小时就能完成,我们之前根本没有这种能力。因此,算力也是一个重要因素。
I want to emphasize that training is the computational intensive problem here. Inferences are much simpler by comparison and here you see the rate of growth of performance demand in petaflops days needed to train a series of models here. If you look at training AlphaZero for example requires 1000 petaflops days, roughly a week on the largest computers available in the world.
我想强调的是,人工智能训练带来的问题是密集的算力需求,程序推理变得简单得多。这里展示的是训练人工智能模型的性能需求增长率。以训练 AlphaZero 为例,它需要 1000 pfs-day,也就是说用世界上最大规模的计算机来训练要用上一周。
This speed has been growing actually faster than Moore’s law. So the demand is going up faster than what semiconductors ever produced even in the very best era. We’ve seen 300,000 times increase in compute from training simple models like AlexNet up to AlphaGo Zero and new models like GPT-3 had billions of parameters that need to be set. So the training in the amount of data they have to look at is truly massive. And that’s where the real challenge comes.
这个增长率实际上比摩尔定律还要快。因此,即使在半导体行业最鼎盛的时代,需求的增长速度也比半导体生产的要快。从训练 AlexNet 这样的简单模型到 AlphaGo Zero,以及 GPT-3 等新模型,有数十亿个参数需要进行设定,算力已经增加了 300,000 倍。这里涉及到的数据量是真的非常庞大,也是我们需要克服的挑战。
Moore’s law, the version that Gordon Moore gave in 1975, predicted that semiconductor density would continue to grow quickly and basically double every two years but we began to diverge from that. Really quickly diverge began in around 2000 and then the spread is growing even wider. As Gordon said in the 50th anniversary of the first prediction: no exponential is forever. Moore’s law is not a theorem or something that’s definitely must hold true. It’s an ambition which the industry was able to focus on and keeping tag. If you look at this curve, you’ll notice that for roughly 50 years we drop only a factor of 15 while gaining a factor of more than almost 10,000.
摩尔定律,即戈登摩尔在 1975 年给出的版本,预测半导体密度将继续快速增长,基本上每两年翻一番,但我们开始偏离这一增长速度。偏离在2000 年左右出现,并逐步扩大。戈登在预测后的五十年后曾说道:没有任何的物理事物可以持续成倍改变。当然,摩尔定律不是定理或必须成立的真理,它是半导体行业的一个目标。仔细观察这条曲线,你会注意到在大约 50 年中,我们仅偏离了约 15 倍,但总共增长了近 10,000 倍。
So we’ve largely been able to keep on this curve but we began diverging and when you factor in increasing cost of new fab and new technologies and you see this curve when it’s converted to price per transistor not dropping nearly as fast as it once fell.
所以我们基本上能够维持在这条曲线上,但我们确实开始跟不上了。如果你考虑到新晶圆厂和新技术的成本增加,当它转换为每个晶体管的价格时,你会看到这条曲线的下降速度不像曾经下降的那么快。
We also have faced another problem, which is the end of so-called dennard scaling. Dennard scaling is an observation led by Robert Dennard, the inventor of DRAM that is ubiquitous in computing technology. He observes that as dimensions shrunk so would the voltage and other assonance for example. And that would result in nearly constant power per millimeter of silicon. That meant because of the amount of transistors that were in each millimeter we’re going up dramatically from one generation to the next, that power per computation was actually dropping quite quickly. That really came to a halt around 2007 and you see this red curb which was going up slowly at the beginning between 2000 and 2007 really began to take off. That meant that power was really the key issue and figuring out how to get energy efficiency would become more and more important as these technologies went forward.
我们还面临另一个问题,即所谓的登纳德缩放定律。登纳德缩放定律是由罗伯特·登纳德 领导的一项观察实验,他是DRAM的发明人。据他的观察,随着尺寸缩小,电压和其他共振也会缩小,这将导致每毫米硅的功率几乎恒定。这意味着由于每一毫米中的晶体管数量从一代到下一代急剧增加,每个计算的功率实际上下降得非常快。这在 2007 年左右最为明显,在 2000 年到 2007 年间开始缓慢上升的功耗开始激增。这意味着功耗确实是关键问题,随着这些技术的发展,弄清楚如何获得更高的能源效率将变得越来越重要。
Combine results of this is that we’ve seen a leveling off of unit processor performance, single core performance, after going through a rapid growth in the early period of the industry of roughly 25% a year and then a remarkable period with the introduction of RISC technologies, instructional-level parallelism, of over 50% a year and then a slower period which focused very much on multicore and building on these technologies.
在经历了行业早期每年大约 25% 的增长之后,随着 RISC 技术的引入和指令级并行技术的出现,开始有每年超过 50% 的性能增长。之后我们就迎来了多核时代,专注于在现有技术上进行深耕。
In the last two years, only less than 5% improvement in performance per year. Even if you were to look at multicore designs with the inefficiencies that come about you see that that doesn’t significantly improve things across this.
在过去的两年中,每年的性能提升不到 5%,即使多核设计也没有显着改善能效方面的问题。
And indeed we are in the we are in the era of dark silicon where multicore often slow down or shut off a core to prevent overheating and that overheating comes from power consumption.
事实上,我们正处于半导体寒冬。多核处理器还是会因为担心过热而限制自身的性能。而过热的问题就来自功耗。
So what are we going to do? We’re in this dilemma here where we’ve got a new technology deep learning which seems able to do problems that we never thought we could do quite effectively. But it requires massive amounts of computing power to go forward and at the same time Moore’s law on the end of Dennard Scaling is creating a squeeze on the ability of the industry to do what it relies on for many years, namely just get the next generation of semiconductor technology everything gets faster.
那么我们能做什么呢?我们在这里陷入了两难境地,我们拥有一项新技术,深度学习,它似乎能够高效地解决很多问题,但同时它需要大量的算力才能进步。同时,一边我们有着登纳德缩放定律,一边有着摩尔定律,我们再也不能期待半导体技术的更新迭代能给我们带来飞跃的性能增长。
So we have to think about a new solution. There are three possible directions to go.
因此,我们必须考虑新的解决方案。这里有三个可能的方向。
Software centric mechanisms where we look at improving the efficiency of our software so it makes more efficient use of the hardware, in particular the move to scripting languages such as python for example better dynamically-typed. They make programming very easy but they’re not terribly efficient as you will see in just a second.
以软件为中心的机制。我们着眼于提高软件的效率,以便更有效地利用硬件,特别是脚本语言,例如 python。这些语言让编程变得非常简单,但它们的效率并不高,接下来我会详细解释。
Hardware centric approaches. Can we change the way we think about the architecture of these machines to make them much more efficient? This approach is called domain specific architectures or domain specific accelerator. The idea is to just do a few tasks but to tune the hardware to do those tasks extremely well. We’ve already seen examples of this in graphics for example or modem that’s inside your cell phone. Those are special purpose architectures that use intensive computational techniques but are not general purpose. They are not programmed for arbitrary things. They are not designed to do a range of graphics operations or the operation is required by modem.
以硬件为中心的方法。我们能否改变我们对硬件架构的设计,使它们更加高效?这种方法称为特定领域架构或特定领域加速器。这里的设计思路是让硬件做特定的任务,然后优化要非常好。我们已经在图形处理或手机内的调制解调器中看到了这样的例子。这些使用的是密集计算技术,不是用于通用运算的,这也意味着它们不是设计来做各种各样的运算,它们旨在进行图形操作的安排或调制解调器需要的运算。
And then of course some combinations of these. Can we come up with languages which match to these new domain specific architecture? Domain specific languages which improve the efficiency and let us code a range of applications very effectively.
最后是以上两类的一些结合。我们是否能开发出与这些特定架构相匹配的语言?特定领域语言可以提高效率,让我们非常有效地开发应用程序。
This is a fascinating slide from a paper that was done by Charles Leiserson and his colleagues at MIT and publish on Science called There’s plenty of room at the Top.
这是查理·雷瑟森和他在麻省理工学院的同事完成发表在《科学》杂志上的一篇论文内容。论文名为“顶端有足够的空间”。
What they want to do observe is that software efficiency and the inefficiency of matching software to hardware means that we have lots of opportunity to improve performance. They took admittedly a very simple program, matrix multiply, written initially in python and ran it on an 18 core Intel processor. And simply by rewriting the code from python to C they got a factor of 47 in improvement. Then introducing parallel loops gave them another factor of approximately eight.
他们想要观察的是软件效率,以及软件与硬件匹配过程中带来的低效率,这也意味着我们有很多提高效率的地方。他们在 18 核英特尔处理器上运行了一个用 Python 编写的简单程序。把代码从 Python 重写为 C语言之后,他们就得到了 47 倍的效率改进。引入并行循环后,又有了大约 8 倍的改进。
Then introducing memory optimizations if you’re familiar with large scale metrics multiplied by doing it in blocked fashion you can dramatically improve the ability to use the cashe as effectively and thereby they got another factor a little under 20 from that about 15. And then finally using the vector instructions inside the Intel processor they were able to gain another factor of 10. Overall this final program runs more than 62,000 times faster than the initial python program.
引入内存优化后可以显着提高缓存的使用效率,然后就又能获得15~20倍的效率提高。然后最后使用英特尔处理器内部的向量指令,又能够获得10 倍的改进。总体而言,这个最终程序的运行速度比最初的 Python 程序快62,000 多倍。
Now this is not to say that you would get this for the larger scale programs or all kinds of environments but it’s an example of how much inefficiency is in at least for one simple application. Of course not many performance sensitive things are written in Python but even the improvement from C to the fully parallel version of C that uses SIMD instructions is similar to what you would get if you use the domain specific processor. It is significant just in its onw right. That’s nearly a factor of 100, more than 100, its almost 150.
当然,这并不是说在更大规模的程序或所有环境下我们都可以取得这样的提升,但它是一个很好的例子,至少能说明一个简单的应用程序也有效率改进空间。当然,没有多少性能敏感的程序是用 Python 写的。但从完全并行、使用SIMD 指令的C语言版本程序,它能获得的效率提升类似于特定领域处理器。这已经是很大的性能提升了,这几乎是 100 的因数,超过 100,几乎是 150。
So there’s lots of opportunities here and that’s the key point behind us slide of an observation.
所以提升空间是很多的,这个研究的发现就是如此。
So what are these domain specific architecture? Their architecture is to achieve higher efficiency by telling the architecture the characteristics of the domain.
那么特定领域架构是什么呢?这些架构能让架构掌握特定领域的特征来实现更高的效率。
We’re not trying to do just one application but we’re trying to do a domain of applications like deep learning for example like computer graphics like virtual reality applications. So it’s different from a strict ASIC that is designed to only one function like a modem for example.
我们在做的不只是一个应用程序,而是在尝试做一个应用程序领域,比如深度学习,例如像虚拟现实、图形处理。因此,它不同于ASIC,后者设计仅具有一个功能,就例如调制解调器。
It requires more domain specific knowledge. So we need to have a language which conveys important properties of the application that are hard to deduce if we start with a low level language like C. This is a product of codesign. We design the applications and the domain specific processor together and that’s critical to get these to to work together.
它需要更多特定领域的知识。所以我们需要一种语言来传达应用程序的重要属性,如果我们从像 C 这样的语言开始就很难推断出这些属性。这是协同设计的产物。我们一起设计应用程序和特定领域的处理器,这对于让它们协同工作至关重要。
Notice that these are not going to be things on which we run general purpose applications. It’s not the intention that we take 100 C code. It’s the intention that we take an application design to be run on that particular DSA and we use a domain specific language to convey the information to the application to the processor that it needs to get significant performance improvements.
请注意,这不是用来运行通用软件的。我们的目的不是要能够运行100 个 C 语言程序。我们的目的是让应用程序设计在特定的 DSA 上运行,我们使用特定领域的语言将应用程序的信息传达给处理器,从而获得显着的性能提升。
The key goal here is to achieve higher efficiency both in the use of power and transistors. Remember those are two limiters the rate at which transistor growth is going forward and the issue of power from the lack of Denard scaling. So we’re trying to really improve the efficiency of that.
这里的关键目标是在功率和晶体管方面实现更高的效率。请记住,晶体管增长的速度和登纳德缩放定律是两个限制因素,所以我们正在努力提高效率。
Good news? The good news here is that deep learning is a broadly applicable technology. It’s the new programming model, programming with data rather than writing massive amounts of highly specialized code. Use data to train deep learning model to detect that kind of specialized circumstance in the data.
有什么好消息吗?好消息是深度学习是一种广泛适用的技术。这是一种新的编程模型,使用数据进行编程,而不是编写大量高度专业化的代码,而是使用数据训练深度学习模型来发现数据中的特殊情况。
And so we have a good target domain here. We have applications which are really demanding of massive amounts of performance increase through which we think there are appropriate domain specific architectures.
所以我们有一个很好的目标域,我们有一些真正需要大量性能提升的应用程序,因此我们认为是有合适的特定领域架构的。
It’s important to understand why these domain specific architectures can win in particular there’s no magic here.
我们需要弄明白这些特定领域架构的优势。
People who are familiar with the books Dave Patterson and I co-authored together know that we believe in quantitative analysis in an engineering scientific approach to designing computers. So what makes these domain specific architectures more efficient?
熟悉大卫·帕特森和我合著的书籍的人都知道,在计算机设计上,我们信奉遵循工程学方法论的量化分析。那么是什么让这些特定领域架构更高效呢?
First of all, they use a simple model for parallelism that works in a specific domain and that means they can have less control hardware. So for example we switch from multiple instruction multiple data models in a multicore to a single instruction data model. That means we dramatically improve the energy associated with fetching instructions because now we have to fetch one instruction rather than any instructions.
首先,他们使用一个简单的并行模型,在特定领域工作,这意味着它们可以拥有更少的控制硬件。例如,我们从多核中的多指令多数据模型切换到单指令数据模型。这意味着我们显着提高了与获取指令相关的效率,因为现在我们必须获取一条指令而不是任何指令。
We move to VLIW versus speculative out of order mechanisms, so things that rely on being able to analyze the code better know about dependences and therefore be able to create and structure parallelism at compile time rather than having to do with dynamically runtime.
我们来看看VLIW和推测性乱序机制的对比。现在需要更好处理代码的也能够得知其依附性,因此能够在编译时创建和构建并行性,而不必进行动态运行。
Second we make more effective use of memory bandwidth. We go to user controlled memory system rather than caches. Caches are great except when you have large amounts of data does streaming through them. They’re extremely inefficient that’s not what they meant to do. They are meant to work when the program does repetitive things but it is somewhat in predictable fashion. Here we have repetitive things in a very predictable fashion but very large amounts of data.
其次,我们更有效地利用内存带宽。我们使用用户控制的内存系统而不是缓存。缓存是好东西,但是如果要处理大量数据的话就不会那么好使了,效率极低,缓存不是用来干这事的。缓存旨在在程序执行具有重复性、可预测的操作时发挥作用。这里执行的运算虽然重复性高且可预测,但是数据量是在太大。
So we go to an alternative using prefetching and other techniques to move data into the memory once we get it into the memory within the processor within the domain specific processor. We can then make heavy use of the data before moving it back to the main memory.
那我们就用个别的方式。在我们把数据导入特定领域处理器上的内存之后,我们采用预提取和其他技术手段将数据导入内存中。接着,在我们需要把数据导去主存之前,我们就可以重度使用这些数据。
We eliminate unneeded accuracy. Turns out we need relatively much less accuracy then we do for general purpose computing here. In the case of integer, we need 8-16 bit integers. In the case of floating point, we need 16 to 32 bit not 64-bit large-scale floating point numbers. So we get efficiency thereby making data items smaller and by making the arithmetic operations more efficient.
我们消除了不需要的准确性。事实证明,我们需要的准确度比用于通用计算的准确度要低得多。我们只需要8-16位整数,要16到32位而不是64位的大规模浮点数。因此,我们通过使数据项变得更小而提高效率。
The key is that the domain specific programming model matches the application to the processor. These are not general purpose processor. You are not gonna take a piece of C code and throw it on one of these processors and be happy with the results. They’re designed to match a particular class of applications and that structure is determined by that interface in the domain specific language and the underlining architecture.
关键在于特定领域的编程模型将应用程序与处理器匹配。这些不是通用处理器。你不会把一段 C 代码扔到其中一个处理器上,然后对结果感到满意。它们旨在匹配特定类别的应用程序,并且该结构由领域特定语言中的接口和架构决定。
So this just shows you an example so you get an idea of how were using silicon rather differently in these environments then we would in a traditional processor.
这里我们来看一个例子,以便了解这些处理器与常规处理器的不同之处。
What I’ve done here is taken a first generation TPU-1 the first tensor processing unit from Google but I could take the second or third or fourth the numbers would be very similar. I show you what it looks like it’s a block diagram in terms of what the chip area devoted to. There’s a very large matrix multiply unit that can do a two 56 x 2 56 x 8 bit multiplies and the later ones actually have floating point versions of that multiplying. It has a unified buffer used for local activations of memory buffer, interfaces accumulators, a little bit of controls and interfaces to DRAM.
这里展示是谷歌的第一代 TPU-1 ,当然我也可以采用第二、第三或第四代,但是它们带来的结果是非常相似的。这些看起来像格子一样的图就是芯片各区域的分工。它有一个非常大的矩阵乘法单元,可以执行两个 56 x 2 56 x 8 位乘法,后者实具有浮点版本乘法。它有一个统一的缓冲区,用于本地内存激活。还有接口、累加器、DRAM。
Today that would be high bandwidth DRAMs early on it with DDR3. So if you look at the way in which the area is used. 44% of is used for memory to store temporary results in weights and things been computed. Almost 40% of being used for compute, 15% for the interfaces and 2% for control.
在今天我们使用的是高带宽DRAM,以前可能用的是DDR3。那我们来具体看看这些区域的分工。 44% 用于内存以短时间内存储运算结果。 40% 用于计算,15% 用于接口,2% 用于控件。
Compare that to a single Skylake core from an Intel processor. In that case, 33% as being used for cach. So noticed that we have more memory capacity in the TPU then we have on the Skylake core. In fact if you were to remove the caps from the cache that number because that’s overhead it’s not real data, that number would even be larger. The amount on the Skylake core will probably drop to about 30% also almost 50% more being used for active data.
将其与英特尔的 Skylake架构进行比较。在这种情况下,33% 用于缓存。请注意,我们在 TPU 中拥有比在Skylake 核心上更多的内存容量,事实上,如果移除缓存限制,这个数字甚至会更大。 Skylake 核心上的数量可能会下降到大约 30%,用于活动数据的数量也会增加近 50%。
30% of the area is used for control. That’s because the Skylake core is an out of order dynamic schedule processor like most modern general purpose processors and that requires significantly more area for the control, roughly 15 times more area for control. That control is overhead. It’s energy intensive computation unfortunately the control unit. So it’s also a big power consumer. 21% for compute.
30% 的区域用于控制。这是因为与大多数现代通用处理器一样,Skylake 核心是一个无序的动态调度处理器,需要更多的控制区域,大约是15 倍的区域。这种控制是额外负担。不幸的是,控制单元是能源密集型计算,所以它也是一个能量消耗大户。 21% 用于计算。
So noticed that the big advantage that exists here is the compute areas roughly almost double what it is in a Skylake core. Memory management there’s memory management overhead and finally miscellaneous overhead. so the Skylake core is using a lot more for control a lot less for compute and somewhat less for memory.
这里存在的最大优势是计算区域几乎是 Skylake 核心的两倍。内存管理有内存管理负担,最后是杂项负担。因此,控制占据了Skylake 核心的区域,意味着用于计算的区域更少了,内存也是同理。
So where does this bring us? We’ve come to an interesting time in the computing industry and I just want to conclude by reflecting on this and how saying something about how things are likely to go forward in the future because I think we’re at a real turning point at this point in the history of computing.
那么我们现在处于一个什么位置呢?我们来到了计算行业的一个有趣时期。我想通过分享一些我的个人思考、以及对未来的一些展望结束这场讲演,因为我认为我们正处在计算领域历史的一个转折点。
From 1960s, the introduction of the first real commercial computers, to 1980 we had largely vertically integrated companies.
从 1960 年代第一台真正的商用计算机的出现到 1980 年,市面上的计算机公司基本上都是垂直整合的。
IBM Burroughs Honeywell be early spin outs out of the activity at the university of Pennsylvania that built ENIAC the first electronic computer.
IBM、宝来公司、霍尼韦尔、以及其他参与了宾夕法尼亚大学制造的世界上第一台电子计算机 ENIAC 公司都是垂直整合的公司。
IBM is the perfect example of a vertically integrated company in that period. They did everything, they built around chips they built the round disc’s in fact the West Coast operation of IBM here in California was originally open to do disc technology and the first Winchester discs were built on the West Coast.
IBM 是那个时期垂直整合公司的完美典范。IBM好像无所不能,他们围绕着芯片制造,他们制造了光盘。事实上,IBM 在加利福尼亚的西海岸业务最初就是光盘技术,而第一个温彻斯特光盘就是在西海岸制造出来的。
They built their own processors. The 360, 370 series, etc. After that they build their own operating system they built their own compilers. They even built their own database estate. They built their networking software. In some cases, they even built application program but certainly the core of the system from the fundamental hardware up through the databases OS compilers were all built by IBM. And the driver here was technical concentration. IBM could put together the expertise across these wide set of things, assemble a world-class team and really optimize across the stack in a way that enabled their operating system to do things such as virtual memory long before other commercial activities can do that.
他们还构建了自己的处理器,有360、370系列等等。之后他们开发了自己的操作系统、编译器。他们甚至建立了自己的数据库、自己的网络软件。他们甚至开发了应用程序。可以肯定的是,从基础硬件到数据库、操作系统、编译器等系统核心都是由 IBM 自己构建的。而这里的驱动力是技术的集中。 IBM 可以将这些广泛领域的专业知识整合在一起、组建一个世界一流的团队、并从而优化整个堆栈,使他们的操作系统能够做到虚拟内存这种事,这可要比在其他公司要早得多。
And then the world changed, really changed with the introduction of the personal computer. And the beginning of the micro processors takes off.
接着出现了重大变化——个人电脑的推出和微处理器的崛起。
Then we change from a vertically organized industry to a horizontally organized industry. We had silicon manufacturers. Intel for example doing processors along with AMD and initially several other companies Fairchild and Motorola. We had a company like TSMC arise through silicon foundry making silicon for others. Something that didn’t exist in earlier but really in the late 80s and 90s really began to take off and that enabled other people to build chips for graphics or other other functions outside the processor.
接着这个行业从垂直转变为水平纵向的。我们有专精于做半导体的公司,例如英特尔和 AMD ,最初还有其他几家公司例如仙童半导体和摩托罗拉。台积电也通过代工崛起。这些在早期都是见不到的,但在 80 年代末和 90 年代开始逐渐起步,让我们能够做其它类型的处理器,例如图形处理器等。
But Intel didn’t do everything. Intel did the processors and Microsoft then came along and did OS and compilers on top of that. And oracle companies like Oracle came along and build their applications databases and other applications on top of that. So they became very horizontally organized industry. The key drivers behind this, obviously the introduction of the personal computer.
但是英特尔并没有一家公司包揽所有业务。英特尔专做处理器,然后微软出现了,微软做操作系统和编译器。甲骨文等公司随之出现,并在此基础上构建他们的应用程序数据库和其他应用程序。这个行业就变成了一个纵向发展等行业。这背后的关键驱动因素,显然是个人电脑的出现。
The rise of shrinkwrap software, something a lot of us did not for see coming but really became a crucial driver, which meant that the number of architecture that you could easily support had to be kept fairly small because the software company is doing a shrink wrap software did not want to have to port and and verify that their software work done lots of different architectures.
软件实体销售等兴起也是我们很多人没有预料到的,但它确实成为了一个关键的驱动因素,这意味着必须要限制可支持的架构数量,因为软件公司不想因为架构数量太多而需要进行大量的移植和验证工作。
And of course the rise in the dramatic growth of the general purpose microprocessor. This is the period in which microprocessor replaced all other technologies, including the largest super computer. And I think it happened much faster than we expected by the mid 80s microprocessor put a series dent in the mini computer business and it was struggling by the by the early 90s in the main from business and by the mid 90s to 2000s really taking a bite out of the super computer industry. So even the supercomputer industry converted from customize special architectures into an array of these general purpose microprocessor. They were just far too efficient in terms of cost and performance to be to be ignored.
当然还有通用微处理器的快速增长。这是微处理器取代所有其他技术的时期,包括最大的超级计算机。我认为它发生的速度比我们预期的要快得多,因为 80 年代中期,微处理器对微型计算机业务造成了一系列影响。到 90 年代初主要业务陷入困境,而到 90 年代中期到 2000 年代,它确实夺走了超级计算机行业的一些市场份额。因此,即使是超级计算机行业,也从定制的特殊架构转变为一系列的通用微处理器,它们在成本和性能方面的效率实在是太高了,不容忽视。
Now we’re all of a sudden in a new area where the new era not because general purpose processor is that gonna go completely go away. They going to remain to be important but they’ll be less centric to the drive to the edge to the ferry fastest most important applications with the domain specific processor will begin to play a key role. So rather than perhaps so much a horizontal we will see again a more vertical integration between the people who have the models for deep learning and machine learning systems the people who built the OS and compiler that enabled those to run efficiently train efficiently as well as be deployed in the field.
现在我们突然进入了一个新时代。这并不意味着通用处理器会完全消失,它们仍然很重要,但它们将不是驱动行业发展的主力,能够与软件快速联动的特定领域处理器将会逐渐发挥重大作用。因此,我们接下来或许会看到一个更垂直的行业,会看到拥有深度学习和机器学习模型的开发者,与操作系统和编译器的开发者之间更垂直的整合,使他们的程序能够有效运行、有效地训练以及进入实际使用。
Inference is a critical part is it mean when we deploy these in the field will probably have lots of very specialized processors that do one particular problem. The processor that sits in a camera for example that’s a security camera that’s going to have a very limited used. The key is going to be optimize for power and efficiency in that key use and cost of course. So we see a different kind of integration and Microsoft Google and Apple are all looking at this.
程序推理是一个关键部分,这意味着当我们进行部署时,可能会有很多非常专业的处理器来处理一个特定的问题。例如,位于摄像头中的处理器用途就非常有限。当然,关键是优化功耗和成本。所以我们看到了一种不同的整合方案。微软、谷歌和苹果都在关注这个领域。
The Apple M1 is a perfect example if you look at the Apple M1, it’s a processor designed by apple with a deep understanding of the applications that are likely to run on that processor. So they have a special purpose graphics processor they have a special purpose machine learning domain accelerator on there and then they have multiple cores, but even the cores are not completely homogeneous. Some are slow low power cores, and some are high speed high-performance higher power cores. So we see a completely different design approach with lots more codesign and vertical integration.
例如Apple M1,Apple M1 就是一个完美的例子,它是由 苹果设计的处理器,对苹果电脑上可能运行的程序有着极好的优化。他们有一个专用的图形处理器、专用的机器学习领域加速器、有多个核心。即使是处理器核心也不是完全同质的,有些是功耗低的、比较慢的核心,有些是高性能高功耗的核心。我们看到了一种完全不同的设计方法,有更多的协同设计和垂直整合。
We’re optimizing in a different way than we had in the past and I think this is going to slowly but surely change the entire computer industry, not the general purpose processor will go away and not the companies that make software that runs on multiple machines will completely go away but will have a whole new driver and the driver is created by the dramatic breakthroughs that we seen in deep learning and machine learning. I think this is going to make for a really interesting next 20 years.
我们正在以与过去不同的方式进行优化,这会是一个缓慢的过程,但肯定会改变整个计算机行业。我不是说通用处理器会消失,也不是说做多平台软件的公司将消失。我想说的是,这个行业会有全新的驱动力,由我们在深度学习和机器学习中看到的巨大突破创造的驱动力。我认为这将使未来 20 年变得非常有趣。
Thank you for your kind attention and I’d like to wish the 2021 T-EDGE conference a great success. Thank you.
最后,你耐心地听完我这次演讲。我也预祝 2021 年 T-EDGE 会议取得圆满成功,谢谢。
(本文首发钛媒体App)